While the big personnel issues of recent years are organizations and their resilience in the face of a changing workforce, this is changing to concerns about how automation will affect skilled workers.
In terms of changing workforce issues, the first challenge is the “great shift change,” or “grey2k,” as a generation of expertise and perspective prepares to retire. The second challenge is adapting to their replacements: the Millennial Generation with its ideas about priorities, personal fulfillment and technology. Third is capturing institutional knowledge to buffer the transition from one generation of employees to the next. The fact that these issues are recognized and managed is a compliment to the leadership, planning and execution of the organizations managing this transition.
However, these changes concern executives and managers regarding the continuation of operations. The retiring employee wasn’t a source of concern to the retiring employee; it was an organizational issue related to the pool of employees as a whole, in particular the magnitude of the change in a relatively short time.
Now, this organizational issue is being replaced at the individual level by a topic of interest to all automation professionals: what will happen to my job? In the press of enthusiasm for the automation industry’s opportunity in innovative hardware (drones, robots, etc.) and software (artificial intelligence (AI), smart systems, etc.), there’s an undercurrent of uncertainty. What will happen to my job?
Robots replace drivers, etc.
The popular press has picked up this story in its discussion of autonomous vehicles and their impact on taxi, truck and delivery drivers (via drones). In mining, autonomous trucks are already in use, for example, at Rio Tinto’s innovative mining operations in Northwest Australia.
But wholesale replacement of job categories is another issue, especially when it comes to skilled workforces associated with manufacturing. It’s easy to imagine a team of engineers sharing coffee (beers?) and wondering aloud about their future. Is it possible they'll be replaced by a AI system or a black-box machine learning algorithm? Are they going to be relegated as “the blacksmiths of the 21st century,” as one analyst recently asked, or is automation an opportunity that leads to “the new face of manufacturing,” to quote another. The old joke about the perfect plant run by a dog and a human (the human feeds the dog and the dog keeps the human from touching the controls) is suddenly taking on new meaning.
[pullquote]The extent of angst over the impact of automation is seen in recent comments by CEOs at IBM and Microsoft. When Ginni Rommety of IBM responds to concerns about IBM’s Watson AI systems—the star of TV ads with Bob Dylan and Serena Williams—with talking points about “new collar” jobs and accelerating rather than replacing employee efforts, that is not a coincidence.
Similarly, Satya Nadella of Microsoft now “gets the message of AI’s downside on jobs” by noting that, “We’re trying to teach machines to learn so that they can do things that humans do, but in turn, help humans,” and publishing a list of six “AI Musts.” (It only took Isaac Asimov three.)
Despite these pauses for recognition among industry leaders that modern technologies will certainly impact the workforce, the march forward continues. SAP announces its Watson competitor, Leonardo; Siemens announces Watson's integration with its Mindsphere platform; and the popularity of Amazon’s Echo AI system in tens of millions of homes each prove future automation and technology developments aren’t slowing down.
The obvious conclusion regarding the next generation of automation and its impact on automation jobs is that it won’t end with this article, this year, or even this decade. It will instead form another geologic layer—like networking, data storage and computing power before it—to be recorded in a future history of automation.
However, process automation professionals can gain much solace if they look at the history of automation technologies, current trends in end user empowerment via innovative software, and the ease of the migration path forward. In reviewing these contexts to imagine what happens next, the logical conclusion is innovation means new opportunities for process automation professionals and their organizations.
A sense of scope
Worldwide annual spending on operational technology (OT), plant and equipment is about $1 trillion dollars. In comparison, annual IT spending is about $4 trillion. A first thought might be that IT must be “bigger” than OT, but that’s misleading, because OT investments typically have 20- to 30-year lifespans, sometimes longer. In IT, a 25-year-old laptop would be a Compaq 386SX “luggable.”
Therefore, regardless of the depreciation method chosen, the installed base for plant equipment amounts to many trillions of dollars spanning industry and technology eras. So when there's excitement about a new set of tools or technology for analytics and insights into production data, the installed base where these innovations are expected to be applied is incredibly diverse in age, vendor, industry and function.
At the same time, consider the many waves of software innovation introduced over the past 30 years to improve production outcomes. These may be focused, like SPC, or a whole new category of software, like APC, or maybe some other acronym. Despite what's included in this historical list, what isn’t seen in the history of these software offerings is a big winner—a broadly accepted product redefining the market in terms of general use. The only exception might be PC-based HMI, but this was just a different way to visualize a process, not a smart system that could automate a person out of a job.
[sidebar id =1]
Innovations need expert guidance
So, is machine learning or deep learning or artificial intelligence—whatever flavor of cognitive computing is currently in favor—going to change this history or repeat it? If we pull apart the value chain associated with analytics, we see three distinct layers of value:
- First, there is the data itself, which must be assembled, modified, cleansed, organized, modeled etc.
- Second, there's the training or prioritization of what insights need to be gleaned from the data. Training, in this case, doesn't refer to supervised or unsupervised approaches to machine learning; it instead means providing feedback into what the analytics are generating to steer it toward important insights.
- Third, there is the analytics itself, the black box executing the algorithms and providing feedback.
The added value introduced in this model by a process automation professional is clearly in the first two stages, making sure the right data is available (stage one) and asking the right questions (stage two). Who is going to have the context and provide insights about what data is relevant and how it should be used? The process automation professional is the expert who can guide the data analytics solution to the right conclusions.
[sidebar id =2]
To train data analytic systems without context is wasted energy, and this is what we’ve seen in business outcomes when a single machine or smart learning software solution is turned loose on process data. It takes the expertise of a process automation professional in the middle to land the value of advances in algorithms in a complex, multi-trillion-dollar installed base like the process industries.
Where innovations in machine learning and other cognitive computing systems are likely to make the greatest impact as self-learning systems is on large groups of homogenous assets. A windmill is pretty much a windmill, regardless of manufacturer or location, so there's a lot an automated system can “learn” on its own by comparing data from a large number of assets.
Further, a data science maxim states "the most important factor in improved outcomes is more data." So, the more assets to work against, the better the systems can predict what's normal and what changes can be used to predict future events, such as degradations in performance or failures. By adding contextual data, such as cost of parts and service personnel, an optimized maintenance program can be created. Human intervention is often required to optimize insights, but much can be accomplished automatically.
Innovations in cognitive computing will change the analytics landscape, but they won't replace the plant and process expertise provided by the process automation professional. They haven't in the past, and the diversity and constantly changing state of process plants will preclude a general solution fitting every problem.
Process automation professionals—the only employees with the required expertise, experience and education—will continue to be the vital link between innovations and outcomes.
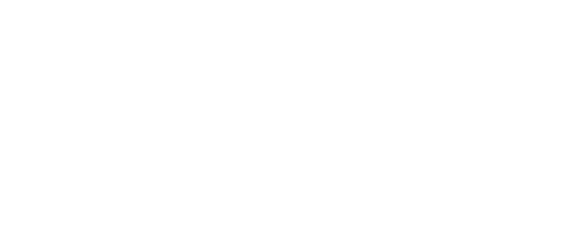
Leaders relevant to this article: