By Jim Montague
It takes plenty of raw materials and energy to make rubber for tires but managing all those feedstocks and handling all that energy can be changeable and difficult. For example, Goodyear Tire & Rubber Co.'s largest U.S. chemical plant in Beaumont, Texas, produces huge amounts of rubber and C5 high-purity isoprene, but it had been facing ongoing hurdles in its high-purity monomer recovery unit, according to Kaylynn Johnston, Goodyear Chemical's senior engineer.
"We have fluctuations in feed composition, and this leads to variability in our operations, which means we may need to compensate with changes in temperature or other setpoints," says Johnston. "The Beaumont plant also is Goodyear's largest energy consumer, and our C5 refinery and its distillation columns are the plant's largest energy consumer, mainly due to the all the steam they use. Because of these challenges, we thought advanced process control (APC) could help."
Johnston and Jon Cimino, of Emerson sales representative Scallon Controls, presented "Sustaining and Extending Advanced Control Value in an Operating Plant" at Emerson Process Management's recent Global Users Exchange 2010.
Johnston reports that the C5 refinery's main operating challenges include:
- A product purification unit that involves multiple distillation trains with 11 columns, both conventional and extractive;
- Ultra-high purity product specifications that require very tight quality controls;
- Multiple large, 200+ tray columns that result in extremely long time constants;
- Different feedstock suppliers with different qualities;.
- Large energy demands and complex production requirements; and
- Safety margins required to compensate for disturbances in feed quality.
As part of a DeltaV migration project at the Beaumont plant, Goodyear also implemented Emerson's SmartProcess distillation optimizer, which uses embedded DeltaV APC, and has on-line key performance indicator (KPI) calculations. SmartProcess includes reusable applications that can be pre-engineered and used multiple times. They include a combination of DeltaV composite blocks, modules and templates, APC tools like PredictPro and Neural, DeltaV graphics, documentation tools, sample configurations, and a simple dynamic simulator. The project also included Emerson's APC Consulting Services, functional designs, control performance audits and implementation assistance.
First Project: Balancing Distillation
The initial phase only covered the first column in the series, to gradually give the plant's staff experience with APC technology, nurture operator acceptance, and show APC's value to Goodyear's management. APC will be added in later phases and other C5 columns by Goodyear's engineers themselves with support from Emerson's APC consultants.
"At first, the C5 refinery's operators were uncomfortable with APC, but the engineers explained its strategic value to them, and this helped their comfort level," says Cimino. "It also helped as the operators got more run-time experience with the APC tools. The dynamic simulator in SmartProcess was helpful, too. For example, we wanted to know ahead of time about controller loading issues, and the simulation showed us that controller loading wouldn't be a problem."
Goodyear also used SmartProcess' Distillation Control Module, which includes standard distillation calculations, module library, predict block and pre-configured neural blocks.
"The first column in the C5 refinery separates chemical components based on different boiling points. Tray temperatures reflect composition on that tray, but they need to be compensated for pressure. As a result, control strategies are based on ‘what comes in must go out,' and so we need material balance of overhead and feed ratio and an energy balance of reflux and feed ratio," explains Johnston. (Figure 1)
To optimize the distillation column, Johnston and her colleagues use SmartProcess' function blocks to set objectives for manipulated variables (MVs), controlled variables (CVs) and constraints for overall objective functions, and then view them all in the software's optimizer window. "We were able to set minimums or maximums for critical variable, and also prioritize those variables," adds Johnston. "We also could establish target setpoints for the column, and calculate setpoint for multiple variables."
When Goodyear turned its newly optimized controllers on, it immediately gained positive results. "Because the column consumes so much energy, Goodyear's engineers wanted to minimize steam use and reduce the amount of product going out of the overhead," adds Johnson. "Once it was optimized, the controller immediately started reducing distillate rate and overhead (OH) concentration. Average product loss via OH was approximately 22%. Product impurities were within specifications, and there were new opportunities to reduce safety margin. And, we reduced overall steam use by 7%."
Second Project: Optimizing Extraction
The second project's challenge was to optimize the C5 column's extractive distillation system for isoprene, which involves four variables. "The temperature setpoint needs to be such that the sidedraw contains most of the impurities, but not too much product," says Johnston. "We first need good information for our manipulated variables, and then we can run tests in manual or automatic." (Figure 2)
These process variables include MVs, CVs, disturbance variables (DVs) and constraints:
- MVs consist of controller setpoints written to by the model-predictive controller (MPC). For example, they can include first-column, side-draw temperature setpoints.
- CVs include process variables to be maintained at a specific value or set point, such as column OH pressure.
- DVs are measured variables that may also affect the value of controlled variables, such as solvent concentration, feed flowrate, reflux remperatures and first-column OH pressure.
- Constraints (LV) are variables that must be kept within an operating range, such as product stream impurity level and percent of product in the impurity stream.
Models were developed for the four process variables, and Goodyear's engineers used their own knowledge to adjust the models' parameters. Actual process data were then used to validate the models.
Johnston said the modeling and tuning resulted in improved temperature control, further reduced product loss, and improved impurity levels. "The new control scheme in our second project also reduced the rate and the product concentration in the purge stream. Overhead product quality was not negatively impacted. We reduced product losses by 7,000 pounds per day. And we reduced energy consumption a moderate amount," adds Johnston. (Figure 3)
To maintain its new APC tools and system in the future, Goodyear may need to change the limit ranges of its manipulated, controlled or constraint variables if feed rate or composition change significantly. "We may also need to tweak or rebuild our models at some point, and we might need to regenerate the controller to adjust performance versus robustness, such as in ‘penalty on move' versus ‘penalty on error' situations," she concludes.
Goodyear plans to install SmartProcess Distillation module and APC models on its additional distillation columns in the C5 refinery process unit, and explore the potential for using APC in other units on non-distillation applications.
Jim Montague is Control's executive editor.
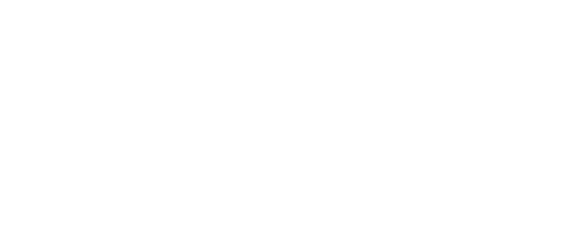
Leaders relevant to this article: