As the digital transformation of the process industries advances, innovative solutions are easing OEE pain points and making way for more flexible methods of applying OEE on an enterprise level. To learn more about how digital transformation is allowing manufacturers to extract new value from this tried and true metric, Keith Larson is joined by Joe Reckamp, principal analytics engineer for Seeq.
Transcript
Keith Larson: Overall equipment effectiveness, or OEE, is tracked in every major manufacturing industry, as it provides insight into key availability, performance and quality parameters that enable teams to make profitable process improvements. But common obstacles, including insufficient data collection or lack of OEE calculation standards across sites, really have caused this key performance indicator's popularity to really wax and wane over the years. Fortunately, as the digital transformation of the process industries advances, innovative solutions are easing these pain points and making way for more flexible methods of applying OEE on an enterprise level.
Hello, my name is Keith Larson, publisher of Control magazine and ControlGlobal.com, and you're listening to a special Solution Spotlight edition of our Control Amplified podcast, sponsored this week by Seeq. To learn more about how digital transformation is allowing manufacturers to extract new value from this tried and true metric, I'm pleased to be joined today by Joe Reckamp, principal analytics engineer for Seeq.
Welcome, Joe, and a real pleasure to talk with you today.
Joe Reckamp: Pleasure to be here. Thanks for having me.
Larson: You bet. Before we jump into the deep end of the new and improved OEE, maybe you can review for us the essential parameters that play into the basic calculation of overall equipment effectiveness?
Reckamp: Yeah, absolutely. So, generally speaking, the standard is a times p times q, which is availability times your performance times your quality percentages. And so, availability is looking at, kind of, your downtime events, some of these maybe planned portions of time, such as when you're cleaning up from a batch or setting up for a new batch. But also, you're focused on, kind of like, the unplanned portions of this time when you're actually trying to produce products, but you have some sort of unplanned stoppages that's occurring. The performance is really looking at how much have you produced in your uptime, and with respect to the maximum possible rate you could be producing, compared to that actual amount you've produced. And then, the quality is how much is of acceptable quality of that product that you've created? So, how much did you actually produce of it that you can sell versus how much might be rejected for some variety of different reasons? Then taking the three of those percentages and multiplying them up together, gives you that kind of overall standard OEE metric.
Larson: So, 100% being the theoretical maximum you can get out of this production line and where you actually stand as a subset of that.
Reckamp: Exactly.
Larson: So, manufacturers have really long used that basic equation to prioritize process improvement projects to address production losses when production is suboptimal, obviously, and really measure the financial impact of process changes, but it's effective application implementation has often been less than straightforward. Can you talk a little bit about some typical obstacles that make OEE measurement itself not as straightforward and cumbersome?
Reckamp: Yeah, absolutely. So oftentimes, companies have that data already that they need for the OEE calculation. However, most OEE solutions have a hard time connecting to all that data. Really, to have that holistic view of OEE, you really need to have data from the process historian, the MES system, and typically limb systems for the quality metrics. But most of the OEE solutions can only connect to one of those at a time. Oftentimes, they're on, for instance, the MES systems. They only have access to that particular data, and so they're limited in kind of the information that they can actually provide to the end users. Some systems actually provide their own sensors try to calculate OEE, but those result in additional capital projects to install the sensors, you end up duplicating your data that you have, which is kind of rejecting that philosophy of having one version, single source of truth that you might desire. Similarly, a lot of OEE solutions are site based. So, they're one site of the time, each site has its own system, and they've got some sort of customization that they've typically done on that site-by-site basis, which makes it hard to get that enterprise view of the system. If you wanted to look at, where can I improve my supply chain or things like that, they're often actually decently large infrastructure projects to put in an OEE solution, which means that a lot of companies just don't even bother for the smaller sites, if they're not producing enough product. It doesn't make enough financial sense to actually do that for those particular sites. And if you really look at just across the enterprises, and across almost every company, there's some point typically in every OEE process that has a manual Excel spreadsheet that provides some sort of tedious process that people have to do on a day-to-day basis, which is just not a fun job to be managing those spreadsheets.
Larson: Yeah, that makes a lot of sense. And I think the fleet point is especially salient because most companies, they have typical pieces of the same types of pieces of equipment, and across dozens, if not hundreds of manufacturing sites and a real lost opportunity not be able to compare that across a fleet basis really, really makes sense.
Reckamp: Yeah, exactly.
Larson: But nowadays, we've got a digital transformation and the industrial IoT. And I think it's, in a lot of ways, upped the ante in terms of what we all expect from our control and visualization systems. We don't just want metrics on performance, but actual insights as well, that will help us improve that performance. Isn't this true of what we expect of our OEE solutions as well nowadays?
Reckamp: Yeah, absolutely. So, as you mentioned, the metric of OEE itself is not actionable. It's just a percentage, right? It's just telling you, what is that percentage of effectiveness that you're looking at? What you really care about is the depth of that information that goes into that percentage. So, what are all those downtime events and their reason codes? And which ones are causing more and or less of an impact on your process? And where can you prioritize those actual continuous improvement projects that you might be looking at? In addition, there's metrics beyond OEE, right? Like, OEE is not an end all be all. It's one metric that provides a view of your business. But you may want higher-level metrics that are looking at supply chain type things, as well as lower level metrics underneath OEE, that give you more details on the actual parts of the process that can be impacted by the operators. So, things like operational availability, or looking at bottlenecks in the process, reject rates, mean time between failure. There's a variety of different calculations that you can do to slice and dice at that OEE calculation underneath the scenes to improve those different areas of availability, performance and quality to end up improving your overall OEE metric in a lower-level way to kind of have it be more actionable for the operators.
Larson: Yeah, that makes a lot of sense. Certainly, I've been following Seeq for many years, and as a leading supplier of those advanced analytics solutions, for us, non-statisticians, you're certainly well positioned to add a new layer of depth and utility to the OEE KPI. Can you explain the new sorts of benefits or gains, when you layer that statistical analysis and advanced analytics on to the more fundamental OEE calculations.
Reckamp: Yeah, there's a variety of different benefits that you get, starting with connecting to the data itself. So, as I mentioned before, you often aren't able to get all the data, but these advanced analytics applications allow you to connect to all that data. So Bring together your historian, your limbs, and your MES data all into one spot to get the holistic view of OEE. You typically have an enterprise view, because you've got more of a SAAS cloud-based platform that allows you to compare and contrast the different sites and use that for, sort of, supply chain planning, if you need to know where could you have additional capacity to make more products, or where can investments be made at different sites around the world.
You also get the micro stoppages. So oftentimes, there are many events that are happening in your process that aren't worth recording if you're trying to do things manually, because they are, you know, seconds long, or even sub seconds long, but they add up over time. So, this often adds up to 20, or more, percent of downtimes. And with these advanced analytics applications, you can look at other data in the process and try to automatically assign reason codes to those events. So, it doesn't add the burden to the operator to actually record what the reason for these micro stoppages was, but gives you that additional information on top of your current metrics.
We talked about other metrics that you may want to calculate, these advanced analytics applications have flexible calculation engines that enable other metrics to be calculated and added into your OEE dashboards and things like that. You can obviously analyze the data, like when you think about what are these advanced analytics applications meant for, they're taking the OEE data and slicing and dicing it in different ways to find out, what are your most prevalent downtime reasons? What are some trends that you might be looking at over time, where you may want to put machine learning applications or algorithms on top of the data to see, is there some sort of trend that these algorithms can actually identify as well? And then often Seeq and advanced analytics applications are really the first place that engineers go to investigate any sort of issue. So, as soon as you have something that you want to go look for, where could I improve this? Or what sort of issue is this? That's going to be the first place they're going to look to say, why did this downtime or issue occur? And that's much easier if you can line up the OEE downtimes, and things like that, with all your other process data and analytics that you might already have.
Larson: That makes a lot of sense, and beats using Excel, too.
Reckamp: Absolutely.
Larson: Are there some specific user case studies that maybe you can share in terms of here's a situation where X chemical company was able to apply it to this problem? Are there are a few that you can share to bring that to life on behalf of our listeners?
Reckamp: Yeah, I've got a couple examples that I can walk through here.
So, one example is a customer was having trouble identifying when a particular failure event was becoming more common. So, they were expecting to see some sort of intermittent failures every once in a while this particular failure mode would take place, because you often see that with any sort of piece of equipment, but they wanted to know when the prevalence of this failure event started increasing so that they could notify the operators to take some sort of action because they have an action that they could take to reduce the issue back to its intermittent state. But it does take away from the manufacturing, so they don't want to do it too often, until that issue starts occurring more often there. And that kind of avoids their product defects that might occur if they didn't take this impact there. And so, they were able to put Seeq actually on top of their current OEE solution, and use the analytics to look at things like mean time between failure and the prevalence of that event occurring, and bring those issues to light in real time, and ended up seeing a generation of millions of dollars of additional product that could be produced every single year at the site.
Larson: It makes sense. Because, as you're operating equipment, it's easy to say, "oh, we just have one of those stoppages," but how do you keep track of how often they're occurring they're part of regular daily life. I imagine this kind of tool would really help find that optimum point where, okay, you've reached the point where you need to stop and clean that out or take some intervention, that makes a lot of sense.
Reckamp: Exactly.
And then another example, we talked a little bit about micro stoppages earlier. Now, we had a customer that had never looked at the micro stoppages before. It wasn't something in their day-to-day lifecycle for the OEE system that they had. And so, they decided to replace that OEE system with Seeq, and found by looking at these micro stoppages, that they were missing about 30% of their downtime that was coming from those micro stoppages themselves.
Larson: That's a big, big point.
Reckamp: And so, they were able to actually use the historian tags and their details of like, what was happening in the process at the time to automate those reason codes as to what was happening based on you know, alarms that were going off at the same time or process context of, you know, they're transitioning to a new product or something like that, and assign those reason codes to those micro stoppages to give more information about what's actually happening in the process without overburdening the operators with additional events and things that they have to deal with. And they also found that some of those micro stoppages are actually avoidable issues that they just never knew that they needed to do something about that they were able to eliminate and improve their processes
Larson: So overall, what would you say to kind of wrap things up? What are the key benefits to be gained by taking traditional OEE calculations and upgrading them to add advanced analytics? What are the key benefits to be gained by looking at it from a holistic perspective across an organization?
Reckamp: Yeah, ultimately, it should impact the business's bottom line. So, it should be a higher percentage should give you a more profitable enterprise. However, there are some considerations in terms of could the business actually sell those additional products if they were making more? Or could they make some sort of other cost savings measure that would kind of save the money that they might have spent otherwise.
But in addition to just hitting the bottom line, there's other things that come into place there. So, there's prioritization of continuous improvement projects that you might have. So, figuring out, where should you be spending your time and investments to improve the process and have the biggest impact overall? We talked about getting that enterprise view and sharing data between sites. You get shared learnings as well. Like you said, there's going to be places where you're going to be producing the same product around the world, and you may be able to identify different improvement projects, or things that could impact both sites rather than just a single site at one time, or just ideas for how they deal with reducing certain reason codes that might be happening at their site.
And then finally, it's like supply chain planning. So oftentimes, as you go into the next year, you're planning for how much product do you need to produce to meet the market demand? And you might need to produce an additional 10%, 20% of product and you can look at where could you fit that in based on the current metrics and information you have, as well as visibility if there's any sort of downstream and upstream facilities where you're maybe making the first step of a product at one facility and moving it to another step. This will give them, sort of, real time visibility into any schedule impacts that they might have that they may not have gotten before.
Larson: So, if you look at this one production step, if you up your OEE there, are you able to accommodate that increased capacity, one step down the road? So you have to look at that OEE as well, I suppose.
Reckamp: Exactly, and vice versa. If you run into an issue at the first site, they may want to know about that downstream, and they may not get that information in real time without that.
Larson: Yeah, well, that makes a ton of sense. Supply chain is the word nowadays. That's certainly one of the one of the key things everybody's looking at. And it's good to have that more more holistic view, and it's interesting that OEE can can play a role there as well.
Well, thanks so much for sharing your perspective with us today, Joe. It's been a real pleasure. I learned stuff, that's for sure. And I'm sure our listeners have as well.
Once again, our guest today has been Joe Reckamp, principal analytics engineer with Seeq, and I'm Keith Larson, publisher of Control magazine ControlGlobal.com, and you've been listening to a Control Amplified podcast. Thanks for tuning in, and thanks to Seeq for sponsoring this episode.
Thank you, Joe. Really appreciate it. And for you listeners. If you've enjoyed what you've heard as well and maybe learned something new along the way, go ahead and subscribe. You can find our podcasts anywhere you find your other podcasts, and the full archive of past episodes are at ControlGlobal.com signing off until next time.
For more, tune into Control Amplified: The Process Automation Podcast.
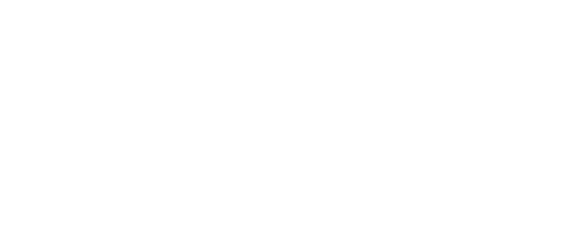
Leaders relevant to this article: